This paper, “Which Economic Tasks are Performed with AI? Evidence from Millions of Claude Conversations,” presents a novel approach to understanding the real-world integration of Artificial Intelligence (AI) into the economy. By analyzing over four million conversations from the Claude.ai platform, the authors provide empirical evidence of how AI is currently being used across various tasks and occupations. They leverage the U.S. Department of Labor’s O*NET Database to map these conversations to specific occupations and tasks, offering a granular view of AI adoption patterns.
The study addresses a critical gap in our understanding of AI’s impact on the labor market. While there’s been extensive speculation and forecasting, systematic empirical data on actual AI usage has been lacking. Existing methods, such as predictive models and surveys, struggle to capture the dynamic interplay between rapidly advancing AI capabilities and their practical application in the workforce. This research bridges that gap by directly examining real-world AI interactions.
The authors introduce a framework to measure the amount of AI usage for tasks across the economy. They map conversations from Claude.ai to occupational categories in the U.S. Department of Labor’s O*NET Database to surface current usage patterns. The approach provides an automated, granular, and empirically grounded methodology for tracking AI’s evolving role in the economy.
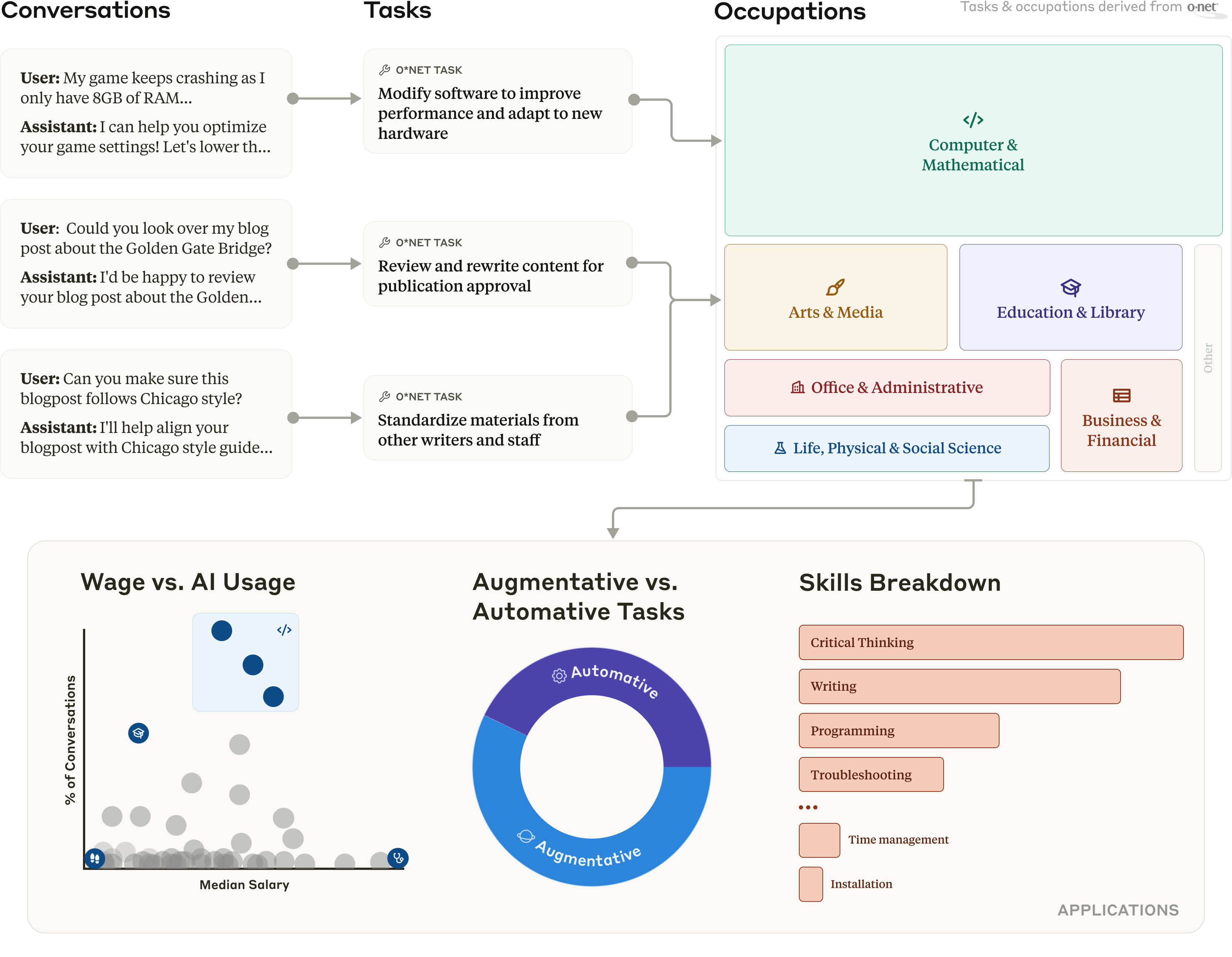
The research makes several key contributions:
- Mapping AI Usage Across Tasks: The study provides a large-scale empirical measurement of AI usage across different tasks in the economy. It reveals that AI is most heavily used in tasks associated with software development and writing-intensive professions. Specifically software engineers, data scientists, bioinformatics technicians, technical writers, and copywriters showed a higher use. Conversely, roles involving physical manipulation of the environment, such as anesthesiologists and construction workers, exhibit minimal AI use.
- Quantifying Depth of AI Integration: The research quantifies how deeply AI is integrated within different occupations. While only a small fraction (around 4%) of occupations use AI for the majority (75% or more) of their tasks, a more substantial portion (around 36%) use it for at least 25% of their tasks. This suggests that AI is beginning to permeate task portfolios across a significant part of the workforce, but deep, pervasive integration remains relatively rare. The AI is used for some tasks within their jobs but not all.
- Identifying Skills in Human-AI Conversations: The study identifies which occupational skills are most prominent in human-AI interactions. Cognitive skills such as reading comprehension, writing, and critical thinking are highly represented, while physical skills (e.g., equipment maintenance) and managerial skills (e.g., negotiation) are less common. This highlights the current complementarity between human capabilities and AI, with AI primarily augmenting cognitive tasks.
- Analyzing Correlation with Wage and Barrier to Entry: The analysis examines the relationship between AI usage, wages, and barriers to entry. AI use is highest in occupations within the upper quartile of wages, particularly those in the software industry, but declines at both the high and low ends of the wage spectrum. A similar pattern emerges for barriers to entry, with peak usage in occupations requiring considerable preparation, such as a bachelor’s degree, rather than minimal or extensive training.
- Assessing Automation vs. Augmentation: The study investigates whether people primarily use AI to automate tasks or augment their capabilities. It finds that augmentation is slightly more common (57% of interactions) than automation (43%), with most occupations exhibiting a mix of both. This indicates that AI serves both as an efficiency tool and as a collaborative partner.
The methodology employed in this research is based on the framework named Clio, a privacy-preserving analysis tool that leverages AI models to extract aggregated insights from millions of human-model conversations. The authors use Clio to classify conversations across occupational tasks, skills, and interaction patterns. The analysis draws from conversation data collected during December 2024 and January 2025. Due to the large number of O*NET task descriptions they created a hierarchical tree of tasks using Clio to classify the conversations.
The O*NET Database and Task Analysis
The O*NET database, a cornerstone of this research, provides a standardized framework for describing occupations and their associated tasks and skills. It acts like a detailed encyclopedia of the U.S. labor market. The challenge, however, is the sheer volume of information – nearly 20,000 unique task statements. To address this, the authors developed a hierarchical tree of tasks, allowing for efficient classification of conversations. This hierarchical approach is like organizing a library: you start with broad categories (e.g., “Science”), then narrow down to specific subcategories (e.g., “Biology”), and finally to individual books (e.g., “Molecular Biology”).
The analysis reveals that computer-related tasks see the largest amount of AI usage (37.2% of all queries), followed by writing tasks in educational and communication contexts.
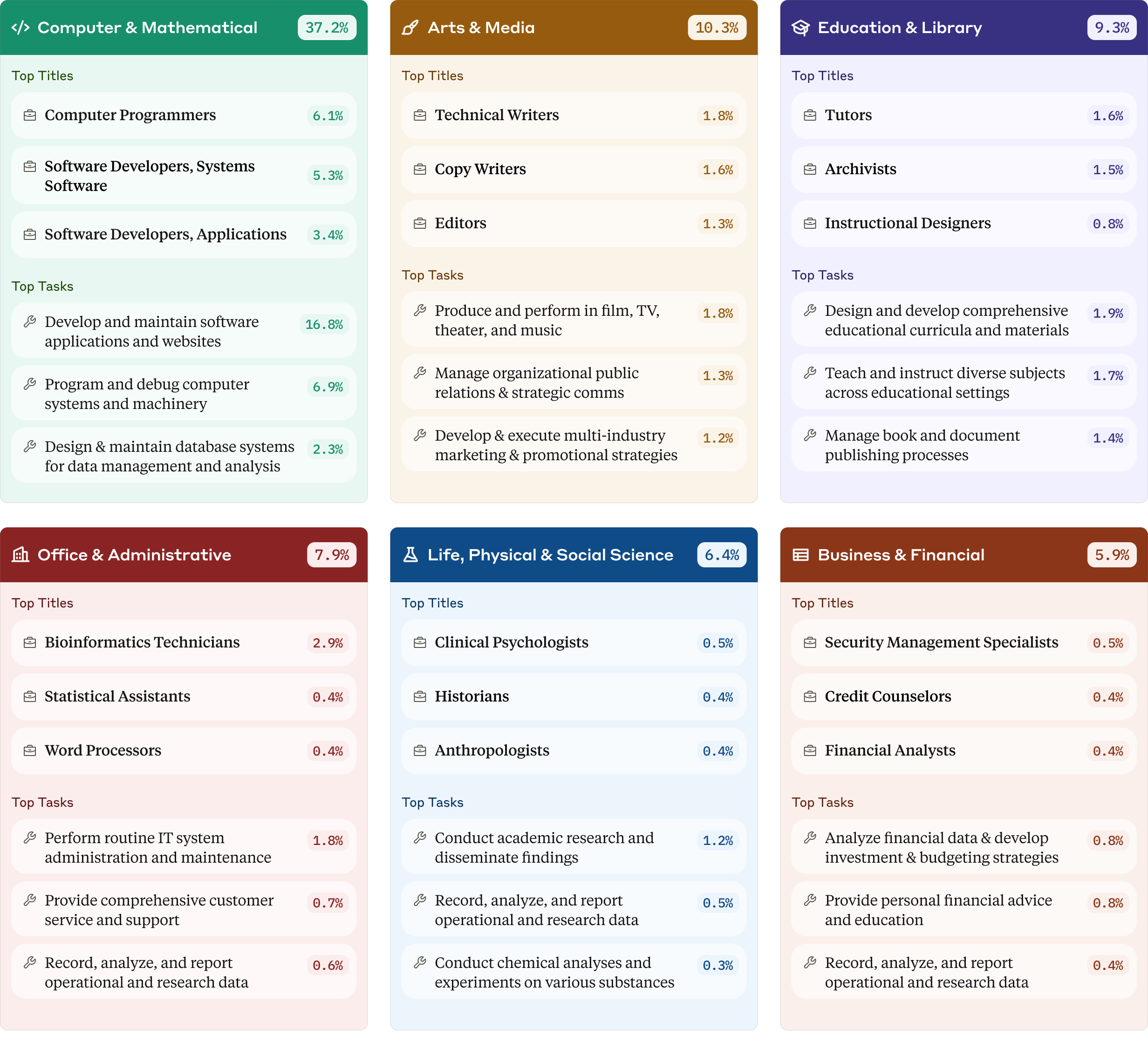
Occupations requiring physical labor had the least amount of AI use.
The authors also looked at how many tasks per occupation show AI usage. Only around 4% of occupations use AI for at least 75% of their tasks. For example, foreign language teachers use AI for collaborating with colleagues on teaching issues and planning course content, but not for writing grant proposals or maintaining student records. In another example, around 36% of occupations show usage in at least 25% of their tasks. For instance, physical therapists use AI for research and patient education but not for hands-on treatment.
The framework also allows determining the occupational skills exhibited during the conversations. For example, skills requiring physical interaction showed the lowest prevalence in Claude.ai traffic, in contrast, cognitive skills had the highest prevalence.
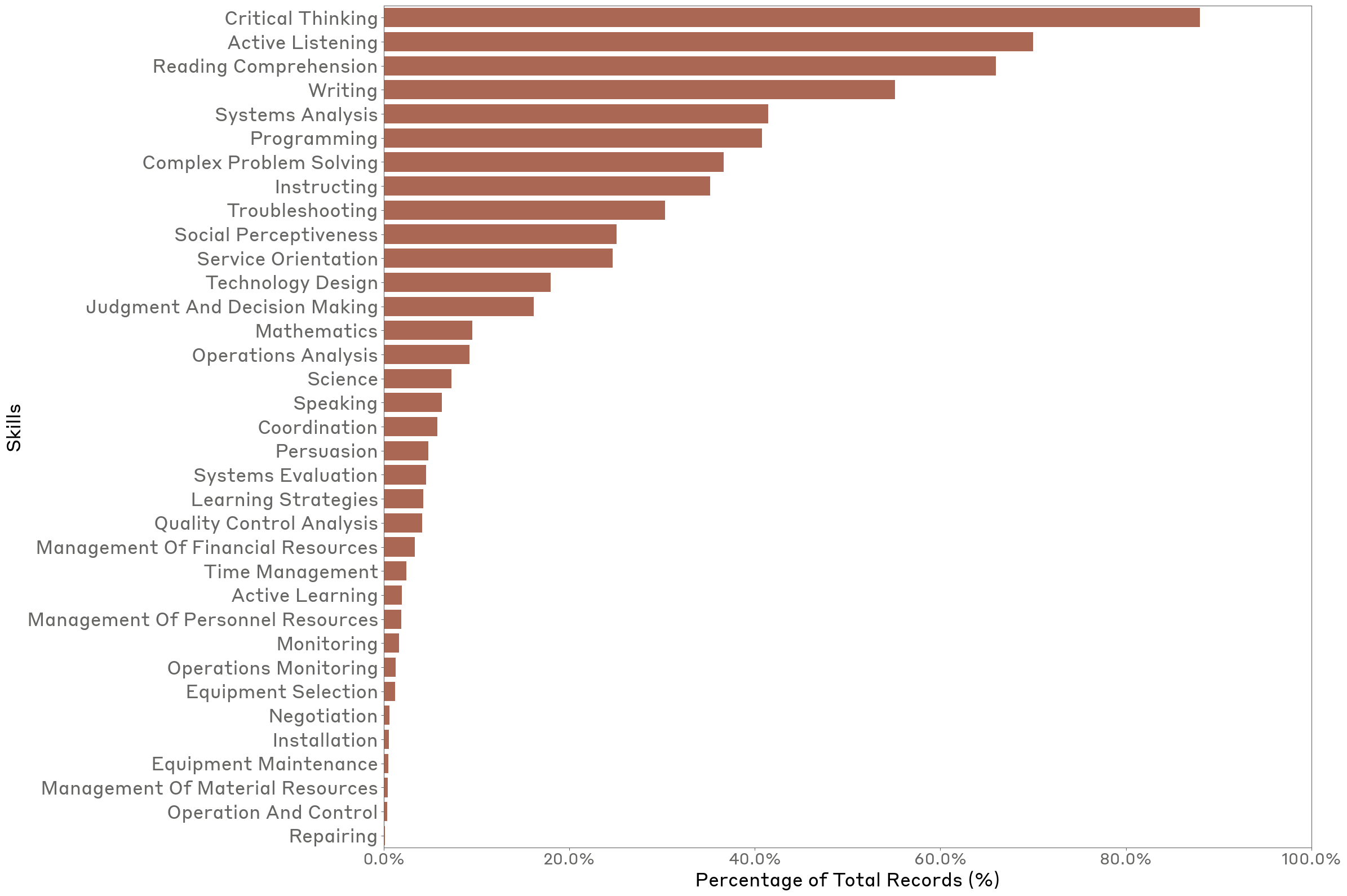
The analysis also reveals that AI use peaks in the upper quartile of wages with computational occupations, while occupations at both extremes of the wage scale show lower usage.
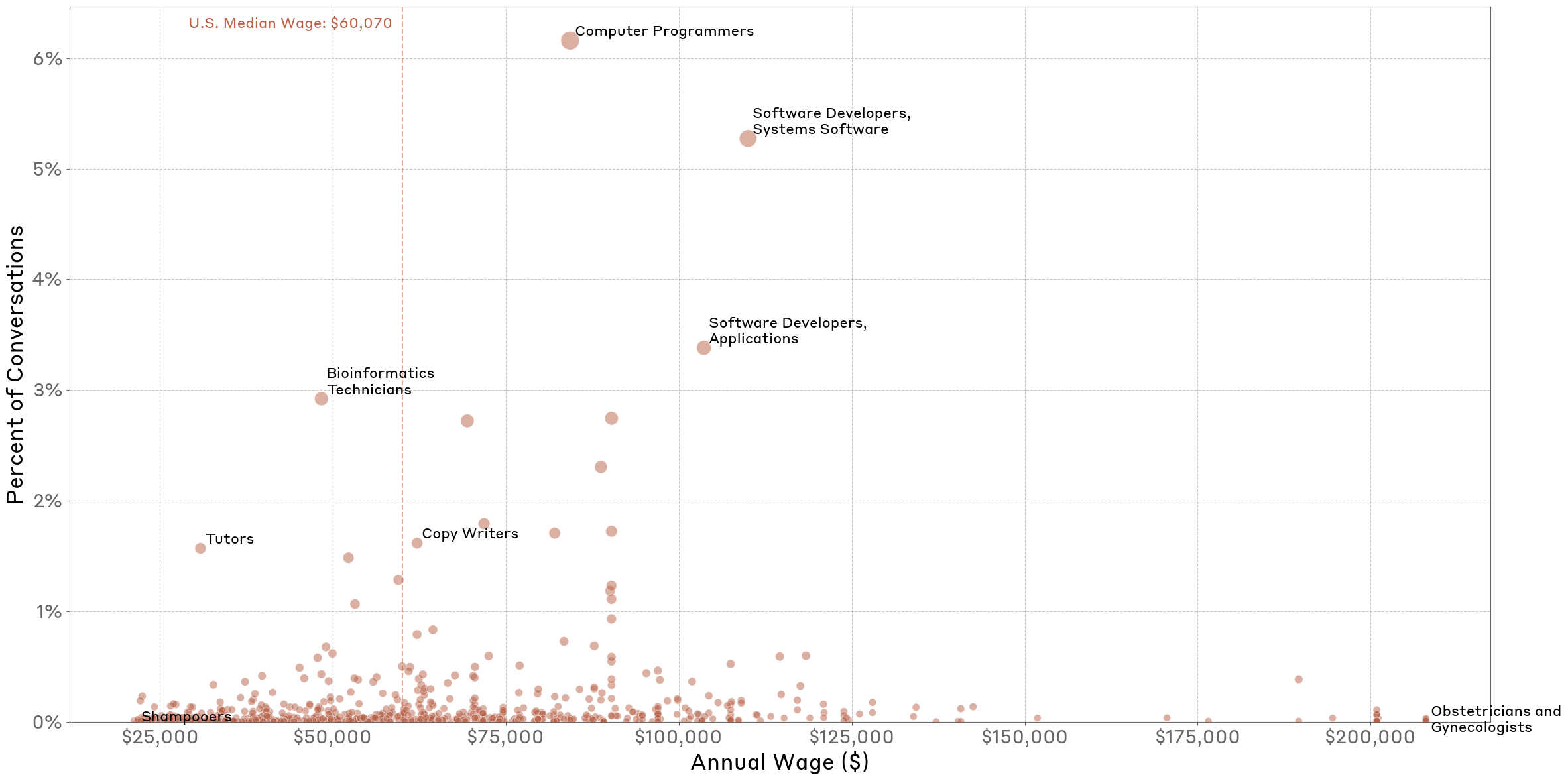
In most of the interactions, users exhibit more augmentative than automative behaviors. Automative behaviors were focused on writing, business, and schoolwork tasks. Augmentative behaviors included front-end development and professional communication tasks.

Finally, the research shows that different models are used for different tasks. Claude 3 Opus sees higher usage for creative and educational work while Claude 3.5 Sonnet is preferred for coding and software development tasks.
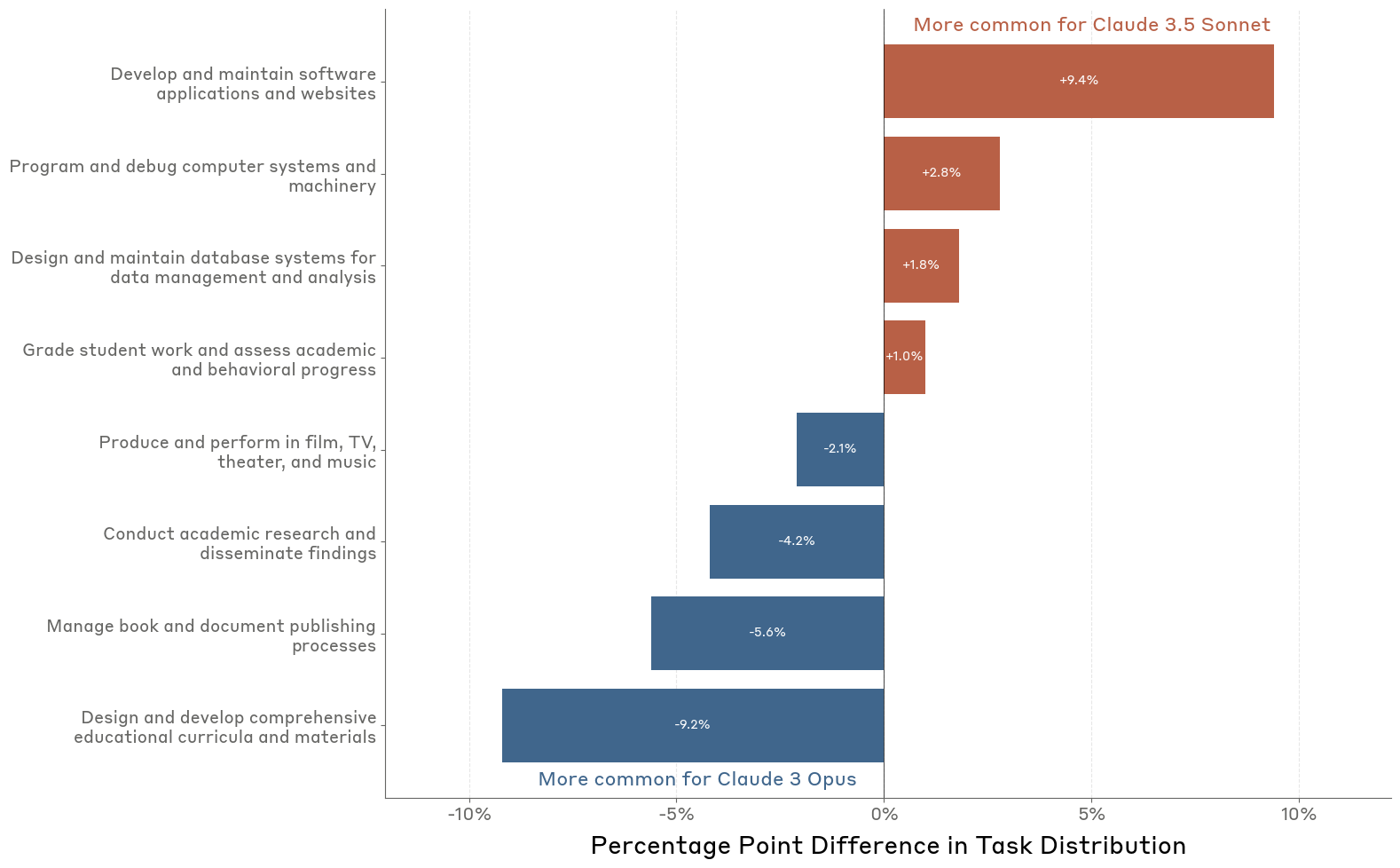
Limitations and Future Work
The authors acknowledge several limitations in their study. The data sample is limited to conversations on Claude.ai during a specific time period, and may not be representative of broader AI usage across different platforms or timeframes. The use of AI to classify user conversations may introduce inconsistencies if the model’s understanding of tasks differs from the intended meaning in the O*NET database. Furthermore, the static nature of the O*NET database may not capture emerging tasks and occupations created or transformed by AI. Finally, the study lacks full context into how users are actually using the outputs of Claude.ai conversations.
Despite these limitations, the research provides valuable insights into AI’s integration into the workforce and establishes a framework for systematically tracking its evolving impact. The authors suggest that future work should focus on dynamic tracking of AI usage, task-level measurement, and understanding the distinction between augmentation and automation. Longitudinal analysis tracking both usage patterns and economic outcomes could help reveal the mechanisms by which AI usage drives changes in the workplace.
In conclusion, this paper offers a significant contribution to our understanding of AI’s impact on the economy by providing empirical evidence of real-world AI usage patterns. The authors’ novel framework and findings pave the way for future research and inform policy decisions aimed at shaping a more beneficial future with AI.